One of the many challenges of working in a recently glaciated environment, like the Central Interior of British Columbia, is the accurate identification of 'soil' sample material and its anomalies.
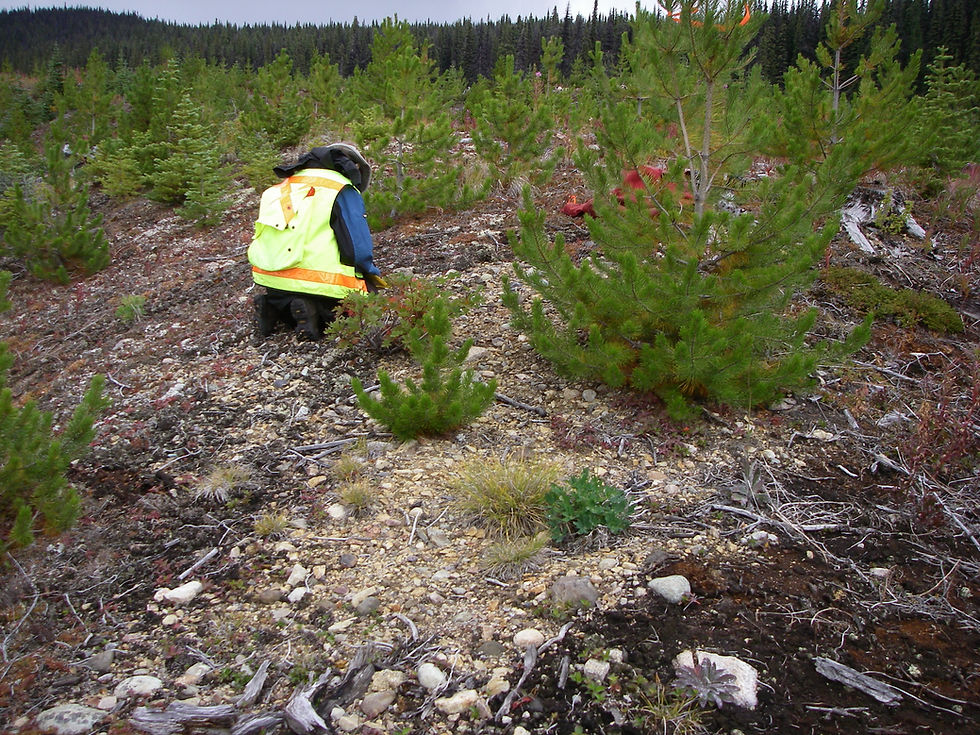
Maybe this sounds familiar?
A soil grid is laid out across promising magnetic signatures, with encouraging geology, and maybe there are even a few historic drill holes or mineral occurrences in the area. With gusto, the team sets out and diligently collects 'B' horizon material from each site and sends their hard work into the lab. Assays are returned, merged with the field data and plotted on a map only to show an array of disconnected 'highs' and 'lows' with very little visual correlation to the fantastic magnetic signatures and geology. What went wrong?
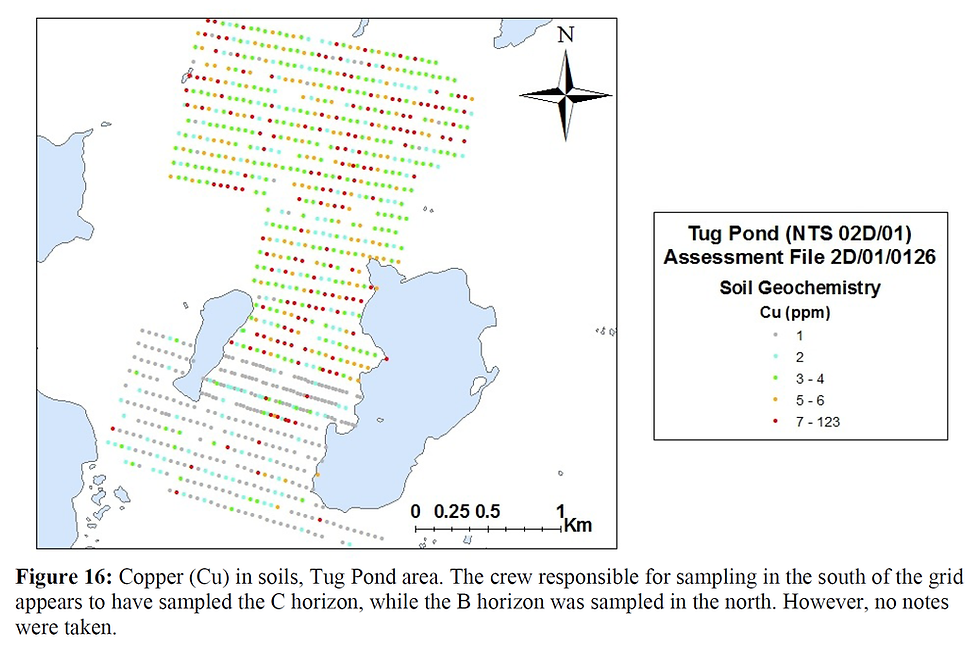
As noted above, in a 2013 CIM short course by Steve Armor at the Geological Survey of Newfoundland and Labrador, one thing that can go wrong is directly comparing different soil horizons.
Other red flags include:
The groupings of sample concentrations or bins -
Are evenly sequential,
The separation of values is likely at the detection limit (1 ppm Cu),
And anything above 6 ppm copper was arbitrarily(?) considered anomalous.
There are samples in the blue waterbodies, which either means the waterbody resolution is too low for the scale, or there may be some muddy and/or organic-rich samples, or beach sand, taken along the shoreline.
The assumption that all values returned at or above the detection limit since the smallest value is 1 ppm copper, but this would likely be described in the text of the report.
The interpretation of the data could be enhanced if the topography, watercourses, and saturated soils (i.e., wetlands) were included as part of the base map.
Since the samples are somewhat evenly spaced, interpretation could be enhanced by interpolation between samples, like inverse distance weighting (IDW), to compare with topography as well as geophysical and geological data.
Lastly, opening up the data with a log-ratio method, and then binning the values by Natural Breaks (or Jenks) would help visually identify patterns related to geology and potential mineralization.
Tip #1 - What colours are your samples?
Textbook definitions of the 'B' horizon will tell you that the presence of clay is the distinguishing feature to differentiate the 'B' horizon from the 'A' and 'C' horizons, but they fail to mention that till, which covers most of the Central Interior of BC, can have a strong clay component. Till is sometimes called 'boulder clay' after all.
Always take photos of the material you are sampling for post-sampling quality analysis.
Soil colour is the result of its mineral composition, element concentration, organic matter, and moisture content. It also reflects the soil properties and soil processes that lead to the creation of the soil. Colour is an important characteristic to determine soil horizon and soil classification.
The 'B' horizon is commonly collected for mineral exploration in Canada. This horizon forms, top-down, by rainwater percolating through the layer(s) above, to precipitate leached material when certain conditions are met. The 'B' horizon may also form in place, by weathering, and can be the result of biological, chemical, or physical actions. The 'B' horizon is typically derived from the 'A' horizon, or mineral layer, which was formed at the surface where all or much of the original structure of the underlying rocks or geological deposits have been obliterated, as well as from the 'O' horizon, which is the detrital layer of organic material accumulated at the surface.
The distinguishing characteristics of the illuvial (top-down) action that forms the 'B' horizon include:
The illuvial concentration of silicate clay, iron, aluminum, humus, carbonates, gypsum, or silica, alone or in combination;
Evidence of removal of carbonates;
The residual concentration of sesquioxides (clays resulting from the chemical weathering of iron/aluminium silicates where they lose all their silicon, and most other ions except iron/aluminium;
Coatings of sesquioxides that make the horizon conspicuously lower in colour value, higher in chroma, or redder in hue, without apparent illuviation of iron, than overlying and underlying horizons;
Alteration which forms silicate clay or liberates oxides, or both, and forms a granular, blocky, or prismatic structure if volume changes accompany changes in moisture content; or
Brittleness.
Alternatively, the 'A' or 'Ah' horizon is collected. This horizon is known as the mineral horizon which has formed at or near the surface and contains less than 17 % organic carbon by weight. The 'h' modifier refers to an increase in accumulated organic matter which is typically represented by a darkening in the soil colour, whereas an 'e' modifier is expressed by a lightening of the soil colour to an ash-grey. The 'Ah' horizon can be useful in exploration for metals, whereas the 'Ae' horizon may assist with exploration that uses indicator minerals; like for diamonds or porphyry deposits.
Below is a cartoon description by Gruškovnjak, L. (2019) that shows:
a) rock parent weathering in situ and, b) soil forming on stratified alluvial sedimentary parent material.
In the Central Interior of BC, we will typically see:
(a) t1 along cliffs and ridges where glaciers and rivers scoured through mountains that have undergone weathering for the past 10,000 years;
(a) t2 in pine flats or terraced benches with or without a very thin 'O' layer that may also be patchy;
a derivative of (a) t3 with a thick 'O' layer in areas that were likely waterbodies not too long ago (geologically speaking);
a derivative of (a) t4 with a thicker 'O' horizon, and much thinner 'A' and 'B' horizons;
or (a) t5 with a thicker 'O' layer of mosses and roots under a canopy of evergreen trees with much thinner 'A', 'Ae' and 'B' horizons.

Gruškovnjak, L. (2019). Hypothetical profile of soil development by top-down pedogenesis. (a) Soil forming on a rock parent material weathering in situ. The weathered rock or saprolite and the soil forming on it constitute the regolith (drawn after models in Schaetzl and Anderson 2005: Fig. 3.2-3; Weil and Brady 2017: Fig. 2.26, 2.36). (b) Soil forming on a stratified alluvial sedimentary parent material (drawn after models in Straffin et al. 1999: Fig. 2; Mandel and Bettis 2001: Fig. 7.1; Holliday 2004: Fig. 5.5; Weil and Brady 2017: Fig. 2.26). Legend: t = time of observation.
Ideally, project-wide soil samples should be within the same colour family, which sounds absolutely insane to a soil scientist, but is one low-tech way to try to ensure that the processes, and source (i.e., top-down soil formation for the 'B' horizon), that formed the soil sample material were similar. In British Columbia, the 'B' horizons are typically variations of brown due to the presence of iron oxides.
If colours are trending towards a bright white, it could mean that the 'Ae' mineral horizon was sampled; particularly if the sample was shallow, or found directly beneath a thick organic layer. If colours are trending towards a pale grey then it's likely the 'C' horizon, where till resides, or a gleysolic soil particularly if it was found in a low-lying area.
As a note, gleysolic soils, which can be grey, are not a desirable sample material because they can develop from a till source and are not necessarily from a bedrock source.
Tip #2 - Map soil sample locations over terrain or hillshade models
Understanding the formation of soil material on different topography can help determine if samples underwent different processes of deposition and accumulation. It's important that different sample media are not compared directly to each other.
For example, 'soil' collected from the slide slope of a ravine with a creek running through will have a quite different formation history than a sample collected from a plateau covered with pine trees. A trail of anomalous 'soil' samples downstream and downslope of mineralized subcrop or outcrop within a ravine can be a misleading series of 'anomalous' samples. These samples are typically colluvial or alluvial in origin. A series of soil anomalies in areas with lower relief (flatter), however, may be more related to low areas in the landscape, with higher organic contents allowing the accumulations of anomalies, such as copper, rather than representing the underlying geology.
Know the formation history of your samples and that there is a difference between soil derived from bedrock, soil from an outcrop on a slope, and soil worked by flood or historical water levels further downslope of the outcrop.
Tip #3 - Re-evaluate anomalies with respect to organic content
One of the greatest influences on metal contents in soils is the presence of organic material. Trace metals tend to bind to organic material within soils as compared to inorganic materials, such as clays and carbonates. The organic material in the soil can preferentially scavenge the metals, causing an abnormal 'high' in metal content when the organic material is dissolved later in the processing of the sample at the lab.
So, if a 'soil' sample is collected within an old stream bed, with a build-up of organic material, or near a stream bank or lake shore within muddy organic layers, the copper content of those samples may appear much greater, to the point of being considered anomalous. These 'anomalies' may instead represent an accumulation over time, rather than an indication of an anomalous source.
Tip #4 - Practice sound statistical methods
Geochemical data are compositional data. Compositional means that the values are represented as proportions where 1 gram per tonne of gold is 1 gram of gold in 1 tonne of sample material, which is the same as 2 grams of gold per 2 tonnes of sample material and so on.
Geochemical data are also often skewed left meaning that there tends to be more results at the smaller values. Using gold as an example again, most of the results will be around the detection limit of the assay method like 0.1 grams per tonne of gold.
The results we receive will look like real numbers, such as 1 or 0.1, but they are ratios and therefore don't meet the requirements of some statistical methods. Most statistics include assumptions that the data can vary independently or have a normal distribution (i.e., normality, where most results are near the middle of a bell curve).
Univariate statistical methods that check one variable, such as average, are typically suitable for geochemical data using a below detection replacement method such as 1/2, 1/3 or the square root of the assay's detection limit.
Bivariate statistical methods, such as scatter plots, and multivariate statistical methods, such as principal component analysis (PCA), are often not suitable without first pre-processing the data. Pre-processing can include imputing (not replacing) below-detection limit values by calculating the expected below-detection limit value based on the other element concentrations, and 'opening' up the data through a log-ratio method on data with all the same units (e.g., converting all values to ppm before normalizing to a log-ratio method).
For example, Pearson Correlation, a bivariate statistical analysis, assumes that there is an absence of outliers and that the scatterplot relationship between each pair of element values is linear, not curved (i.e., linearity).
Tell-tale signs of poor statistical practices include:
Displaying unit measurements in graphs (particularly mixed units like ppm and ppb), scatter plots with logarithmic values on the x and/or y-axis
Hand-drawn lines drawn on graphs,
PCA biplots that are not centred at 0 or show the poor separation of the elements (they are primarily clustered in one direction or quadrant),
Bulls-eye interpretations around one sample,
And values grouped by 10 or some other even number (e.g., 0 - 10, 10 - 20, 20 - 30, etc.).
Tip #5 - Be wary of single-point anomalies
Regardless of your QA/QC program or sample spacing, it is good practice to be skeptical of single-point geochemical anomalies. Ideally, there will be a gradual decrease in value around samples with greater values unless there is a corroborating structural or geological explanation like a fault or change in rock type.
Hand-drawn soil contours will often fall into the trap of single-point anomalies, whereas interpolation methods, such as Inverse Distance Weighting (IDW) for gridded data or Kriging for non-gridded data, will smooth out, or reduce, the effects of single-point anomalies. Most single-point anomalies will be subdued, or removed, with appropriate pre-processing of the data using a log-ratio transformation and Natural Breaks (Jenks optimization) classification (binning method to group similar samples).
TLDR:
Soil sampling in British Columbia doesn't have to be complex if a few simple tips are followed:
Watch for colour changes,
Be wary of anomalies in low-lying areas,
Practice sound statistical methods,
And be cautious following up on single-point anomalies.
Contact us to help you Design, Compile, and Interpret your geochemical and biogeochemical results.
About the Author:
Dr. Diana Benz has over 25 years of experience working in the mineral exploration industry searching for diamonds and metals in a range of roles: from heavy minerals lab technician to till sampler, rig geologist, project manager and business owner/lead consultant. She has a Bachelor of Science in General Biology, a Master of Science in Earth Sciences researching diamond indicator mineral geochemistry, and a PhD in Natural Resources and Environmental Studies using geochemical multivariate statistical analysis techniques to interpret biogeochemical data for mineral exploration. Diana has conducted fieldwork in Canada (BC, NWT, YT and ON) as well as in Greenland. She has also been involved, remotely through a BC-based office, on mineral exploration projects located in South America, Africa, Eurasia, Australia and the Middle East. Currently, Diana is the owner of Takom Exploration Ltd., a small geological and environmental firm focused on metal exploration in BC and the Yukon.
References
Soils of Canada: Identify Horizons
A Concise Guide to Compositional Data Analysis
Linear Analysis Regression Assumptions
Pearson Correlation Assumptions
Introduction to Multivariate Analysis
Alba Giacalone, Antonio Gianguzza, Santino Orecchio, Daniela Piazzese, Gaetano Dongarrà, Salvatore Sciarrino & Daniela Varrica(2005)Metals distribution in the organic and inorganic fractions of soil: a case study on soils from Sicily, Chemical Speciation & Bioavailability,17:3,83-93, DOI: 10.3184/095422905782774892
Comments