Artificial Intelligence Adoption in Mineral Exploration: What to Watch Out For
- Diana M. Benz, Ph.D.
- Oct 28, 2024
- 10 min read
On October 22, 2024, the Government of Canada announced the launch of two new programs aimed at growing Canada's Artificial Intelligence (AI) ecosystem. These initiatives focus on developing generative AI applications and enabling AI adoption among small and medium-sized businesses to boost productivity. This announcement is part of a broader C$2.4 billion package of AI-focused initiatives unveiled in Budget 2024, designed to accelerate job growth, enhance productivity, and ensure responsible AI usage. As artificial intelligence adoption in mineral exploration becomes increasingly prevalent, it's crucial for companies to approach their adoption with a critical eye. To help you navigate this process effectively, here are some key considerations to keep in mind when implementing AI solutions on your mineral exploration projects.
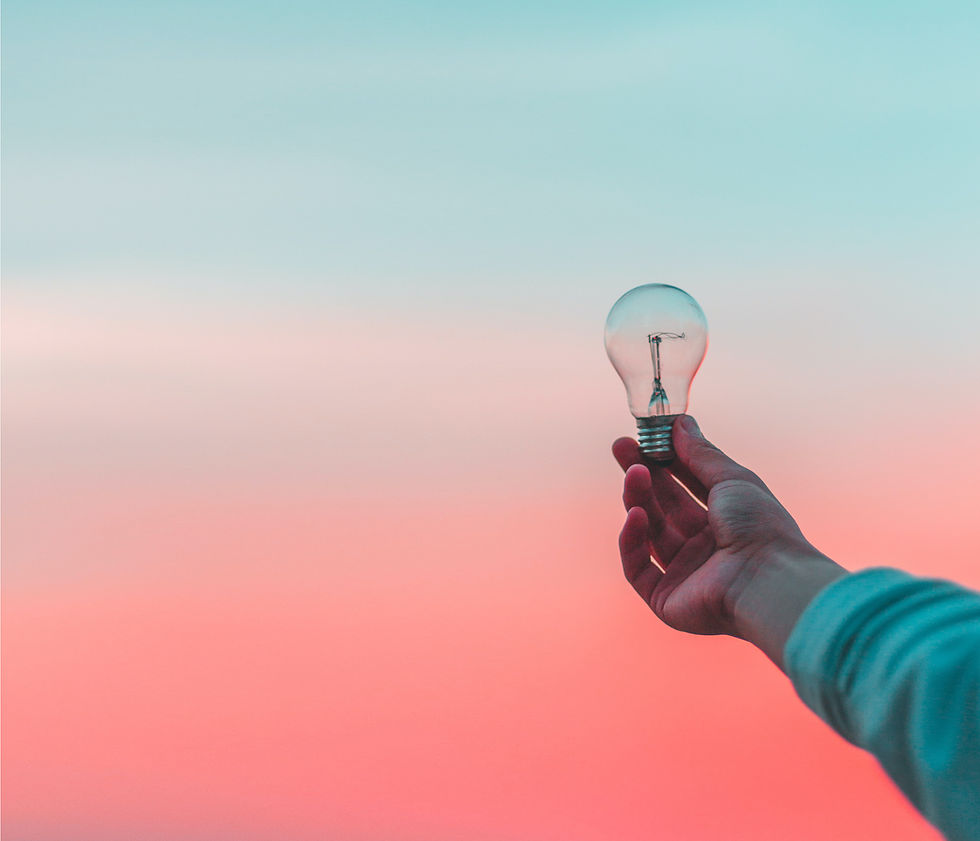
Artificial Intelligence Companies in Canadian Mineral Exploration
While many Artificial Intelligence companies operate in Canada, those specifically focused on mineral exploration are limited. The two notable companies that specialize in AI for mineral exploration and have headquarters in Canada are:
Minerva Intelligence - Based in Vancouver, BC, Minerva develops AI software to assist in mineral exploration and geological mapping.
Kore Geosystems - Headquartered in Toronto, ON, Kore provides AI-driven solutions for mineral exploration and mining operations.
It's important to note that the field of AI in mineral exploration is still developing, and many companies that offer AI solutions for the broader mining industry may not specifically focus on exploration.
Key Considerations When Adopting AI in Mineral Exploration
1. Uniqueness of the Artificial Intelligence Solution
What to watch for:
AI services that are widely available and don't offer a competitive advantage
Example: An AI company advertises a "revolutionary" algorithm for identifying gold deposits. However, upon closer inspection:
Basic model - The AI simply highlights areas with known geological features associated with gold deposits (e.g., certain rock types, fault lines) based on publicly available geological maps by using a standard classification algorithm without considering the complex interplay of factors that lead to gold mineralization.
Tailored model - A more sophisticated AI solution would:
Incorporate multiple data types beyond just geology (e.g., geochemistry, geophysics, historical drilling results, remote sensing, etc.)
Use advanced techniques to identify subtle patterns across these datasets.
Account for the specific geological context of your exploration area.
Provide confidence levels for its predictions and explain the key factors influencing each target area.
Real-world impact: A basic model might simply redraw existing geological maps with a "prospectivity" label, providing little new insight. A tailored model would identify the known promising areas and may locate areas that traditional methods might overlook, potentially leading to new discoveries.
Questions to ask:
How does this AI solution differentiate from others in the market?
What unique value does it bring to our exploration efforts?
What data sources, beyond public geological maps, does the AI use?
Can you explain why specific areas are highlighted, beyond just matching the known favourable rock types?
2. Technical Robustness
What to watch for:
Models that rely too heavily on coarse-scale data
Lack of precise, actionable targets
Absence of error calculations or inappropriate statistical methods
Example: If the AI-generated prospectivity map closely mirrors the regional-scale geological map for the desired deposit type, it may indicate an over-reliance on coarse-scale data. This can result in:
Large, generalized target areas (e.g., 6 km or larger).
Missing potential mineralization events that don't fit the dominant geological models.
Overlooking smaller-scale features that could be significant for mineralization.
Real-world impact: You might miss out on valuable deposits that occur in unexpected geological settings or overlook areas where overlapping mineralization events have occurred.
Questions to ask:
What scales of data does the AI model incorporate?
How precise are the generated targets?
Can you provide error calculations for the model?
What statistical methods are used, particularly for geochemical data?
3. Statistical Methods in Geochemical Data Analysis
What to watch for:
Inappropriate use of Z-scores or other methods that assume a normal distribution
Lack of consideration for the compositional nature of geochemical data
Example 1: Using Z-scores for geochemical data analysis can be problematic because -
Z-scores assume the data have a normal distribution, which is often not the case for geochemical data.
They are sensitive to outliers, which are common in geochemical datasets.
They ignore the compositional nature of geochemical data.
Example 2: Some AI solutions might divide each element's concentration by the median value across all samples. While this may seem like a reasonable way to normalize the data, it's inappropriate for geochemical data because:
It doesn't account for the compositional nature of the data (the data sum to a constant, like 100%).
It can create spurious correlations between elements.
It doesn't preserve the relative magnitudes between different elements, which can be geologically significant.
Instead, log-ratio transformations should be used to properly handle the compositional nature of geochemical data and prepare it for further statistical analyses that typically assume that the data have a normal distribution.
Real-world impact: Inappropriate statistical methods can lead to the misinterpretation of geochemical data. For example, a company using simple correlation analysis on raw geochemical data might incorrectly identify a relationship between gold and arsenic. This could lead to targeting areas with high arsenic content that actually have no gold potential, wasting valuable exploration resources.
Questions to ask:
How does your model handle the compositional nature of geochemical data?
What methods do you use to address non-normal distributions in element concentrations?
How does your AI handle outliers and extreme values common in geochemical datasets?
4. Business Model and Ownership
What to watch for:
Frequent changes in ownership or business model.
Payment structures involving equity or project stakes.
Example: An AI exploration company initially offers its services for equity stakes in client companies. Later, it is acquired by a large corporation, raising concerns about data confidentiality and potential conflicts of interest for its existing clients.
Real-world impact: A mineral exploration company partners with an AI firm that takes equity stakes in projects. Later, the AI firm is acquired by a major mining company. This creates a conflict of interest, as the major mining company now has insider knowledge of multiple junior explorers' projects, potentially compromising the juniors' competitive advantage or negotiating position for future deals.
Questions to ask:
Has the company undergone recent ownership changes?
What is the current payment structure for services?
Are there any requirements for equity or project ownership?
5. Data Quality and Quantity
What to watch for:
Insufficient or low-quality input data or the inability to accommodate areas with sparse data.
Lack of diverse geological settings in the training data.
Overuse of similar or related data layers, especially in geophysical datasets.
Failure to address data redundancy and overlapping information in input data.
Example 1: An AI model trained primarily on data from porphyry copper deposits in the Andes is applied to explore for gold in the Canadian Shield. The model's predictions may be unreliable due to the significant differences in geological settings and mineralization styles.
Example 2: An AI model is given multiple layers of magnetic data that have been processed in different ways (e.g., total magnetic intensity, reduced to pole, first vertical derivative). Without proper data preparation, this can lead to an overemphasis on magnetic features when identifying promising areas. As a result, the model might not effectively learn which specific aspects of the magnetic data are truly important for finding mineral deposits.
Real-world impact: This oversight can result in biased predictions that give too much importance to areas with strong magnetic signatures, potentially missing other important geological clues for mineralization.
Questions to ask:
What type and amount of data is required for optimal model performance?
How does the AI handle areas with limited data?
How do you handle multiple related data layers, especially in geophysical datasets?
What methods do you use to prevent overlapping or redundant information from skewing the model's results?
Can you explain your process for selecting the most relevant features from geophysical data?
6. Model Transparency
What to watch for:
"Black box" solutions that don't explain their methodologies.
Results that can't be validated by geologists.
Example: An AI company claims its model has a success rate of discovering mineralization (or doesn't provide any success rates) but refuses to disclose how this accuracy is calculated or what specific features the model uses to make its predictions, citing proprietary algorithms.
Real-world impact: An AI company provides target areas for gold exploration but doesn't explain how these targets were generated. The exploration company drills these targets without understanding the underlying rationale. When the drilling yields poor results, the exploration company has no way to refine or improve the AI's predictions, leading to a loss of confidence in the technology and a wasted exploration budget.
Questions to ask:
Can you explain how the AI arrives at its conclusions?
How can geologists validate and interpret the results?
7. Integration with Existing Workflows
What to watch for:
AI solutions that don't complement traditional geological methods.
Difficulty in integrating with current exploration processes.
Output formats incompatible with existing software and systems.
Example: An AI company delivers promising target areas based on its analysis, but the outputs are provided in a proprietary format that is not compatible with the exploration company's existing GIS software.
Real-world impact: The exploration company faces several challenges:
Geologists spend excessive time manually converting and reformatting data, undermining the efficiency gains the solution was supposed to provide.
The company may need to invest in additional software or tools to handle the AI outputs, leading to unexpected costs and requiring staff training.
If the outputs are not usable, it could result in delays in decision-making and project timelines.
Questions to ask:
How does this AI solution work alongside traditional exploration methods?
What changes to our current workflows will be necessary?
In what format(s) will the AI outputs be provided?
Are these outputs compatible with our existing GIS software (please specify the software)?
Can you provide outputs in specific formats and projections that we require (list your required formats)?
Is there an additional cost for custom output formats?
How flexible is your system in adapting to our existing data management protocols?
8. Scalability and Customization
What to watch for:
One-size-fits-all solutions that don't adapt to specific project needs.
Inability to scale as a project progresses.
Example: An AI model performs well for early-stage regional targeting but cannot be refined to incorporate new, detailed geophysical data acquired during follow-up surveys, limiting its usefulness as the exploration project progresses.
Real-world impact: An AI model performs well for an early-stage project targeting porphyry copper deposits on a large block of claim encompassing hundreds of square kilometres. However, when the company asks for detailed targeting it fails to provide useful results. The lack of scalability and customization means the company can't leverage its AI investment across different project types or stages, limiting the overall value of the technology to the company.
Questions to ask:
Can the AI be customized for our specific geological settings and targets?
How does the solution scale from early-stage exploration to advanced targeting?
9. Limitations of AI Models Adapted for Geological Data
What to watch for:
Misapplication of general statistical methods or AI models to geological data without proper adaptation.
Overreliance on techniques that may not capture the complex, non-linear nature of geological processes.
Example 1: Advanced Statistical Methods
Many statistical methods used in mineral exploration, such as Principal Component Analysis (PCA), were originally developed for general data analysis. While PCA is a powerful tool for dimensionality reduction and feature extraction, its standard application can be problematic for geochemical data:
Standard PCA assumes linear relationships, which may not hold for complex geological processes.
It doesn't account for the compositional nature of geochemical data (data that sum to a constant, like 100%).
It can be sensitive to outliers, which are common in geochemical datasets.
Steps aren't taken to account for small sample sizes or a poor sample-to-variable ratio.
Geoscientists have developed specialized versions, like RQ-mode PCA, and use log-ratio transformations before applying PCA. However, not all AI solutions in mineral exploration properly implement these adaptations.
Real-world impact: An exploration company using standard PCA on raw geochemical data might misinterpret element associations, leading to incorrect targeting. For example, it might overemphasize correlations between certain elements that are artifacts of the closure effect rather than true geological relationships. This could result in drilling programs that target areas with low potential for mineralization, wasting valuable resources.
Example 2: Convolutional Neural Networks (CNNs)
CNNs, originally developed for image recognition tasks, have been adapted for use in mineral exploration. While powerful, they come with limitations:
CNNs excel at identifying spatial patterns but may struggle with the subtle, gradual changes often present in geological data.
They typically require large amounts of labelled training data, which can be scarce in mineral exploration.
CNNs may overemphasize linear or textural features visible in geophysical or remote sensing imagery, potentially missing important complex relationships in geochemical or other data types.
Real-world impact: An exploration company using a CNN trained on geophysical data to identify potential mineral deposits might focus too heavily on physical features. This could lead to overlooking deeply buried deposits that don't have clear expressions or misinterpreting near-surface features that aren't indicative of mineralization.
Questions to ask:
How have you adapted standard statistical methods and AI models for geological data analysis?
Do you use specialized techniques for compositional data when analyzing geochemical datasets?
Can you explain how your methods account for the non-linear and compositional nature of the geological processes to ensure the AI doesn't overly prioritize linear patterns in the data?
Can you explain how your AI models integrate different data types (e.g., geophysical, geochemical, geological) to make predictions?
How do you validate the predictions of your AI models against geological knowledge and field observations?
Conclusion
As the Canadian government invests heavily in AI adoption, the mineral exploration industry has a unique opportunity to leverage these technologies. However, it's crucial to approach AI adoption with a critical eye, ensuring that the chosen solutions truly add value to your exploration efforts. By carefully evaluating AI service providers, understanding the key considerations for implementation, and being aware of potential pitfalls, mineral exploration companies can harness the power of AI while maintaining the geological expertise and ground-truthing exercises fundamental to successful exploration.
Remember, the goal is to use AI as a powerful tool to augment human expertise, not replace it. With thoughtful implementation, AI can significantly enhance the efficiency and effectiveness of mineral exploration efforts, potentially leading to more thoughtful boots-on-the-ground, more discoveries, and better resource delineation.
About the Author
Dr. Diana Benz has 28 years of experience in the mineral exploration industry searching for diamonds and metals in a range of roles: from heavy minerals lab technician to till sampler, rig geologist, project manager and business owner/lead consultant. She has a Bachelor of Science in General Biology, a Master of Science in Earth Sciences researching diamond indicator mineral geochemistry, and a PhD in Natural Resources and Environmental Studies using geochemical multivariate statistical analysis techniques to interpret biogeochemical data for mineral exploration. Diana has conducted fieldwork in Canada (BC, NWT, YT and ON) as well as in Greenland. She has also been involved, remotely through a BC-based office, in mineral exploration projects in South America, Africa, Eurasia, Australia and the Middle East. Diana owns Takom Exploration Ltd., a boutique geological and environmental firm focused on metal exploration in BC and the Yukon.
Comments